Redesigning an AI Tool Suite
Role: Senior UX Designer (UX Research, UX Design, UX Strategy)
Duration: February 2023 – Present
Tools & Methods: Figma, User Interviews, Heuristic Evaluations, Usability Testing, Design Systems Integration, Rapid Prototyping
Overview
A leading education technology company with a massive publishing library and proprietary data had developed a robust suite of standalone AI tools, including:
- AI Text Generation
- AI Translation
- AI Copy Editing
- AI Image Generation
Despite their impressive capabilities, these AI tools were initially developed “dev-first,” lacking cohesive design standards, brand alignment, and intuitive interaction models. Even expert users struggled to leverage their full potential.
As the Senior UX Designer, I led the effort to revitalize this proprietary AI tool suite with the following objectives:
- Improve Accessibility and Ease of Use
Enable new users to get started with AI-driven content creation while maintaining robust personalization options for power users. - Establish a Design Language and Update the Design System
Incorporate the company’s existing design principles, evolve them to incorporate AI-specific standards, and ensure a scalable, future-proof foundation as we add new models and capabilities. - Plan for Future Interoperability and Integration
Although currently standalone, these tools will eventually fit into a unified AI experience, seamlessly connecting with the company’s broader content and assessment ecosystems. I am designing this upcoming unified experience as a natural evolution of the current standalone tools. - Highlight Reliability and Customization as Differentiators
Leverage our proprietary data sets and strong publishing assets to provide users with trusted, high-quality outputs. Offer granular customization—such as choosing models and specifying source inputs—surpassing industry-standard AI tools.
The Challenge
Legacy Development and User Friction
The tools were built with robust back-end functionalities but lacked front-end user-centered design. Each tool felt siloed, used inconsistent interfaces, and ignored brand guidelines. This created steep learning curves for new users and left even experts frustrated by cumbersome interactions.
Key Pain Points:
- Complexity for Novices: Disconnected features, unclear entry points, and cryptic controls.
- Expert User Fatigue: Lack of shortcuts, personalization, and intuitive navigation.
- Brand Inconsistency: The tools failed to reflect the reliability and educational authority of the company’s established brand and design system.
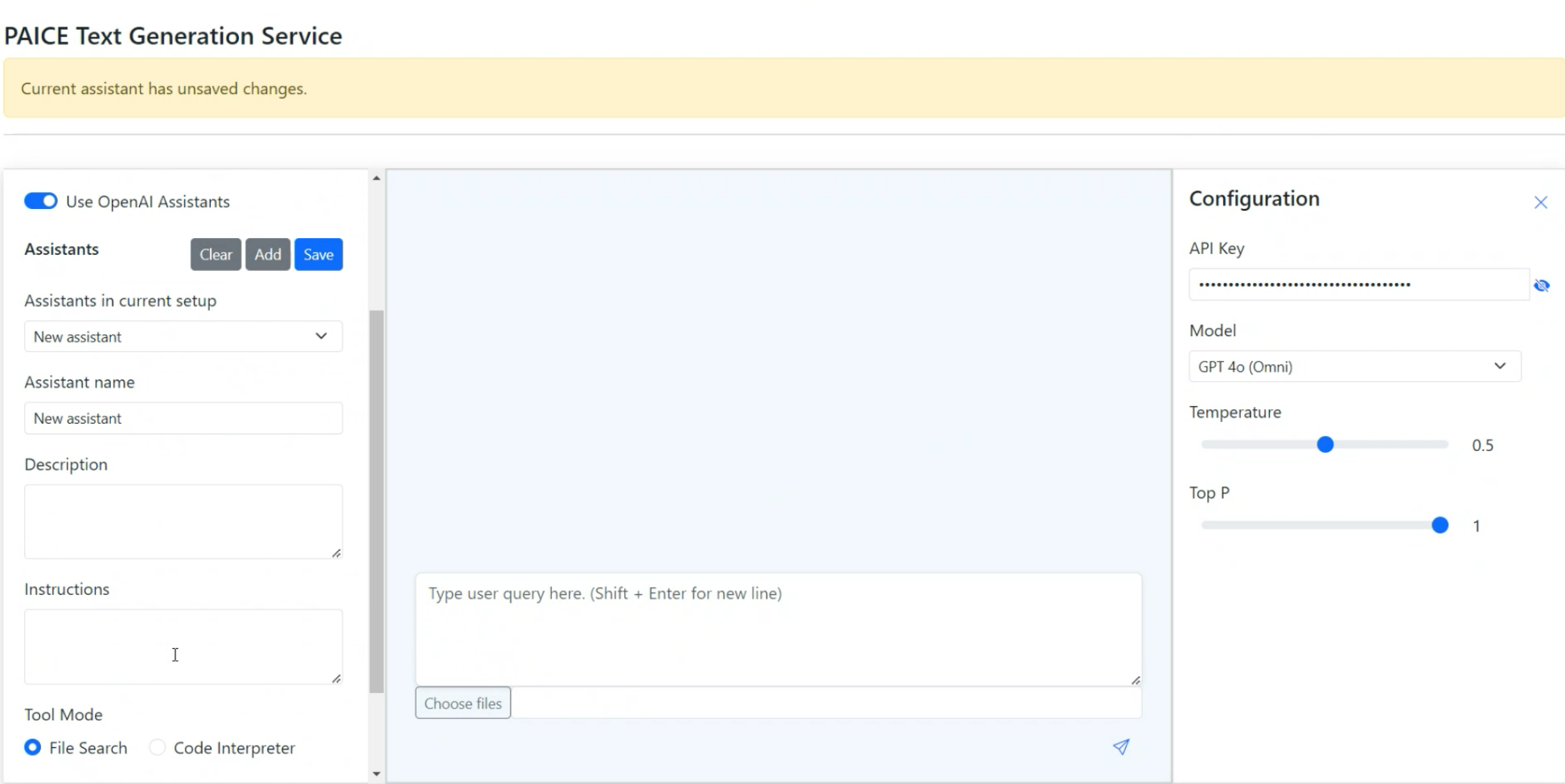

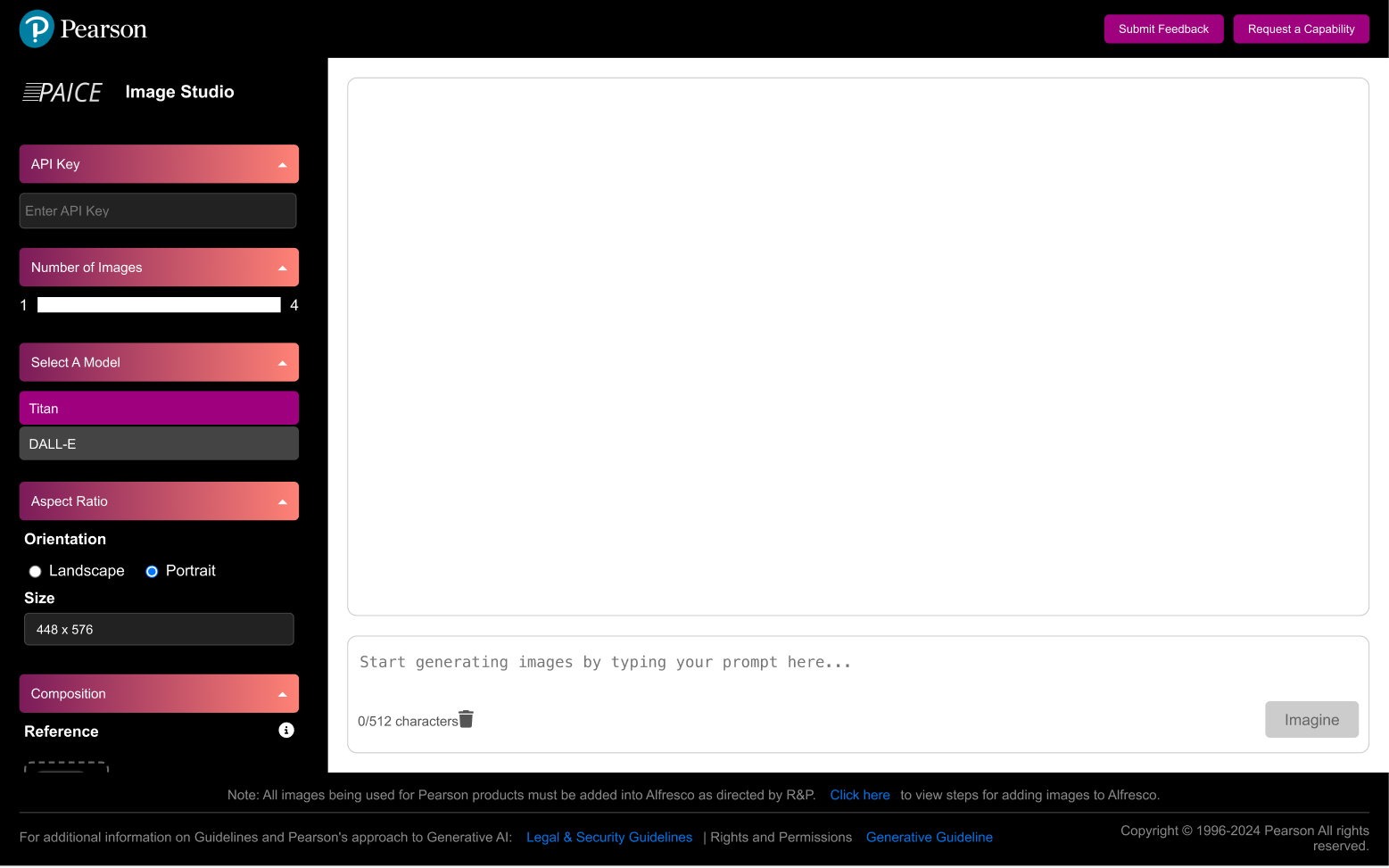
Differentiation in a Saturated AI Market
While AI tools proliferate, few provide deep, reliable, and customizable content generation built on verified academic sources. We needed to highlight our unique data pedigree and provide transparent, trustworthy experiences that distinguish us from generic industry-standard models.
Research & Insights
User Interviews & Contextual Inquiries
I interviewed both novice and expert users (internal content teams, educators, instructional designers) to uncover usability issues and understand their mental models.
Key Findings:
- Need for Guided Onboarding: New users required clear tutorials and discoverable features.
- Demand for Transparency: Users wanted to know the reliability of their AI outputs—cited sources, academic rigor, and relevant publication dates.
- Desire for Customization: Expert users sought granular control, like choosing domain-specific models (e.g., “STEM-focused”) or limiting source material to specific years or academic levels.
Competitive and Heuristic Evaluations
We studied competitor tools to understand standard practices and gaps. Most lack robust customization or clear data provenance. This reaffirmed our differentiation strategy: emphasize data reliability, model-switching, and source specificity to serve education professionals more effectively.
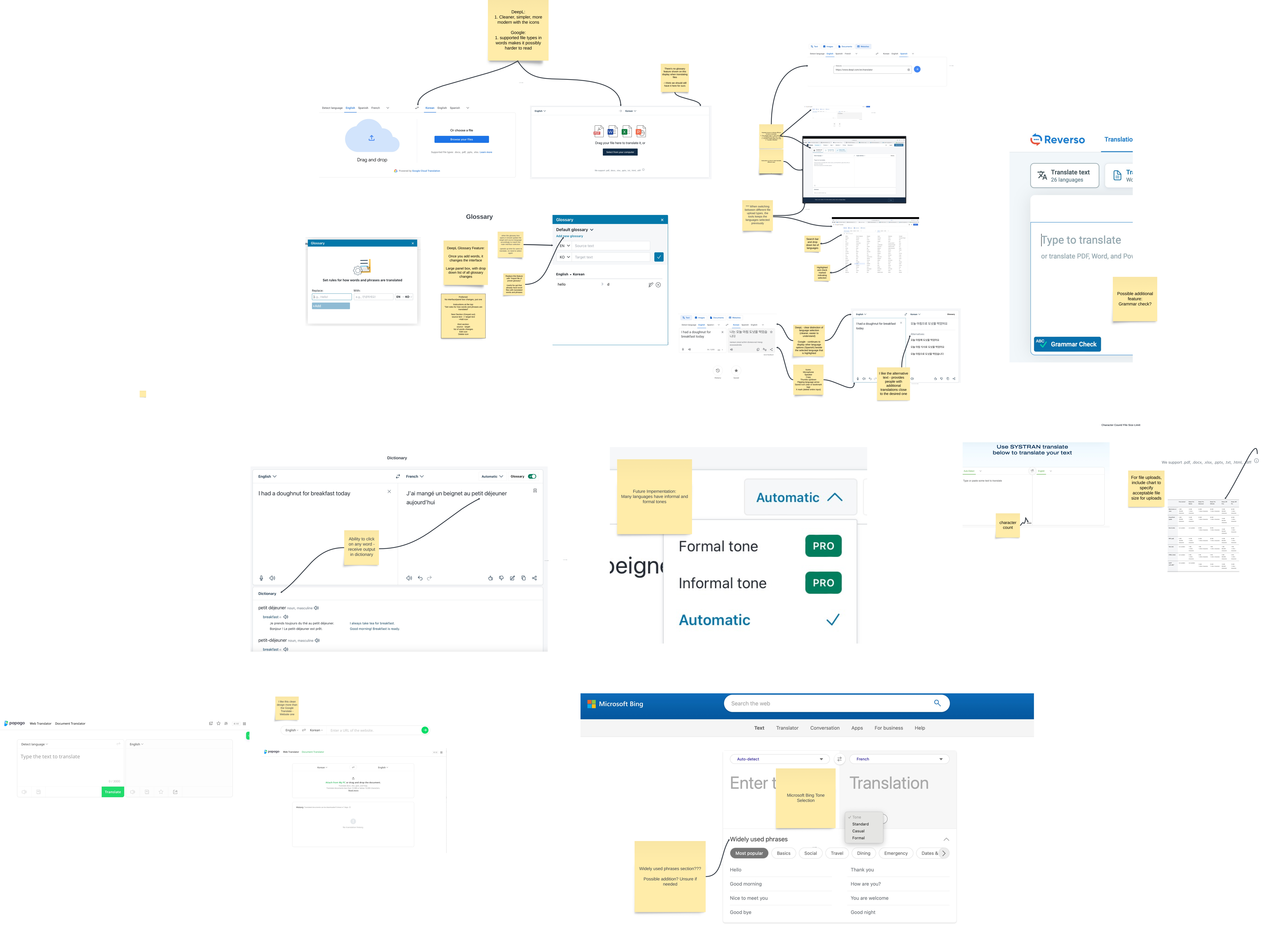
UX Strategy
- Integrating AI Standards into the Design System:
I led an initiative to update our existing design system to include AI-specific components, interaction patterns, and guidelines. This ensures that as we add more AI features or develop future products, the design system scales and maintains brand coherence. - Refined Standalone Interfaces with a Path to Unification:
For current standalone tools, I created a consistent look and feel while planning a future unified AI experience. This v2 unified dashboard is in the works, and the lessons learned from redesigning each standalone tool inform the design of a centralized interface. Ultimately, users will be able to access text, translation, copy editing, and image generation tools from one central AI hub. - Guided Onboarding and Progressive Disclosure:
Introduced onboarding flows, tooltips, and tiered feature sets. New users see essential functions upfront, while experts have easy access to advanced settings (e.g., source filters, model selection) when needed. - Advanced Customization Settings:
Implemented panels allowing users to:- Select from multiple AI models trained on different corpora.
- Filter content sources (e.g., “Use only peer-reviewed journals post-2015,” or “Focus on higher education STEM textbooks”).
- Adjust parameters like creativity levels, tone, and complexity.
- Trust and Transparency Features:
Incorporated visible indicators of data reliability and citations for text generation. Users could see a “data pedigree” of the sources used. This transparency built trust and highlighted our competitive advantage—academic-quality data. Visible data reliability indicators (such as citing source authenticity) bolster user confidence. - Future-Forward Interoperability Planning:
Although not fully implemented yet, I’m also working closely with product managers and engineers to ensure a smooth integration of these AI tools into the company’s content, assessment, and authoring platforms. Early design and prototyping show how AI-driven functions can be accessed directly within these other products, emphasizing seamless interoperability.
.png)
Designing the Experience
Interface Highlights
- Consistent Branding & Visual Hierarchy:
Applied updated design system components to each standalone AI tool, ensuring a cohesive visual language. The refined interfaces reflect the company’s reliable, authoritative brand identity. - Customization Panels:
In each tool, a side panel allows users to refine parameters—choosing models, filtering by source types, adjusting complexity—making advanced settings easily accessible but not overwhelming to beginners. - Trust and Transparency Features:
Integrated indicators showing source provenance. Aligning to the design system also provided assurance to users that they were in a secure internal tool. - Scalable Components for a Future Unified Experience:
The revised layouts, component libraries, and pattern guidelines will streamline the next phase: building a single entry point (the unified AI dashboard) where all tools coexist. The standalone tools now serve as building blocks for this unified vision.



Validation and Iteration
Usability Testing
- Novice Users: Found it simpler to get started thanks to improved signposting, guided tours, and consistent controls.
- Expert Users: Praised the advanced customization options and transparency features. Switching models and specifying data sources reduced trial-and-error and improved content relevance.
Continuous feedback loops allowed iterative refinement of the interface wording, iconography, and complexity levels.
Stakeholder Alignment
Product managers, engineering teams, and data scientists recognized the value of a stable, brand-aligned UI foundation. This foundation will expedite integration efforts into the rest of the product ecosystem and set a standard for future AI-driven tools.
Results and Impact
- Enhanced User Adoption and Efficiency:
A more cohesive, discoverable interface helped new users feel confident faster, and experts achieved their goals with fewer steps. - Design System Advancement:
By introducing AI-specific standards into the design system, we created a scalable reference that will guide future AI feature integrations. This positions the company for rapid growth in AI capabilities without fracturing the user experience. - A Path Toward a Unified AI Experience:
While currently stand-alone, the redesigned tools now share enough DNA to be combined seamlessly in a forthcoming unified AI dashboard. This next step will make it easier for users to transition between tasks (e.g., generating text, translating it, then refining with copy editing) all in one place. - Differentiation through Reliability and Customization:
By emphasizing transparency and allowing fine-grained control over data and models, we set ourselves apart from standard industry AI tools, reinforcing the company’s reputation as a trusted authority in education.
Looking Ahead
- Unified AI Dashboard (v2):
Drawing on the design patterns established in the stand-alone tools, I’m now designing a centralized environment. This will allow users to access all AI tools from one interface, providing a fully integrated and even more powerful workflow. - Expanded Source and Model Options:
Plans include offering domain-specific models and letting users specify even finer-grained source parameters. For instance, users might filter for “peer-reviewed journals in physics published between 2018-2020.” - Continuous Improvement and User Feedback:
As AI models evolve and the product ecosystem grows, we will iterate on the design system to ensure the experience remains intuitive, brand-aligned, and user-centric.
Conclusion
Through strategic UX research, design system updates, and a user-focused approach, we transformed a set of complex, dev-first AI tools into approachable, brand-consistent experiences. This redesign improves accessibility for new users, empowers experts with deeper customization, and paves the way for a future unified AI dashboard.
By leveraging our proprietary educational data, ensuring transparency, and supporting interoperability, we reinforced the company’s unique position in the market. Our work not only solves current pain points but also sets a scalable foundation for ongoing innovation in educational AI.